Nota
Haz clic aquí para descargar el código de ejemplo completo o para ejecutar este ejemplo en tu navegador a través de Binder
Trazar los vectores de soporte en LinearSVC¶
A diferencia de SVC (basado en LIBSVM), LinearSVC (basado en LIBLINEAR) no proporciona los vectores soporte. Este ejemplo demuestra cómo obtener los vectores de soporte en LinearSVC.
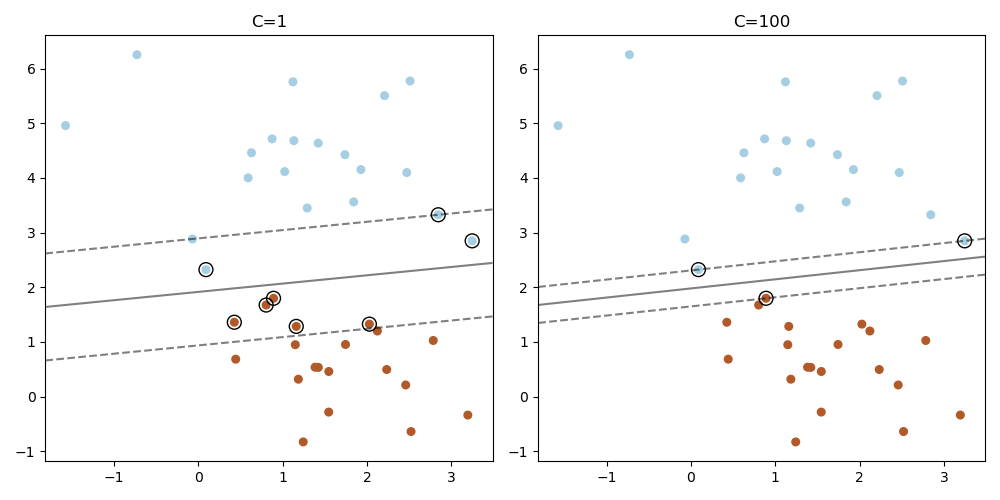
import numpy as np
import matplotlib.pyplot as plt
from sklearn.datasets import make_blobs
from sklearn.svm import LinearSVC
X, y = make_blobs(n_samples=40, centers=2, random_state=0)
plt.figure(figsize=(10, 5))
for i, C in enumerate([1, 100]):
# "hinge" is the standard SVM loss
clf = LinearSVC(C=C, loss="hinge", random_state=42).fit(X, y)
# obtain the support vectors through the decision function
decision_function = clf.decision_function(X)
# we can also calculate the decision function manually
# decision_function = np.dot(X, clf.coef_[0]) + clf.intercept_[0]
# The support vectors are the samples that lie within the margin
# boundaries, whose size is conventionally constrained to 1
support_vector_indices = np.where(
np.abs(decision_function) <= 1 + 1e-15)[0]
support_vectors = X[support_vector_indices]
plt.subplot(1, 2, i + 1)
plt.scatter(X[:, 0], X[:, 1], c=y, s=30, cmap=plt.cm.Paired)
ax = plt.gca()
xlim = ax.get_xlim()
ylim = ax.get_ylim()
xx, yy = np.meshgrid(np.linspace(xlim[0], xlim[1], 50),
np.linspace(ylim[0], ylim[1], 50))
Z = clf.decision_function(np.c_[xx.ravel(), yy.ravel()])
Z = Z.reshape(xx.shape)
plt.contour(xx, yy, Z, colors='k', levels=[-1, 0, 1], alpha=0.5,
linestyles=['--', '-', '--'])
plt.scatter(support_vectors[:, 0], support_vectors[:, 1], s=100,
linewidth=1, facecolors='none', edgecolors='k')
plt.title("C=" + str(C))
plt.tight_layout()
plt.show()
Tiempo total de ejecución del script: (0 minutos 0.223 segundos)