Nota
Haz clic aquí para descargar el código completo del ejemplo o para ejecutar este ejemplo en tu navegador a través de Binder
Almacenamiento en caché de los vecinos más cercanos¶
Este ejemplo demuestra cómo precalcular los k vecinos más cercanos antes de usarlos en KNeighborsClassifier. KNeighborsClassifier puede calcular los vecinos más cercanos internamente, pero precalcularlos puede tener varios beneficios, como un control más fino de los parámetros, el almacenamiento en caché para su uso múltiple, o implementaciones personalizadas.
Aquí utilizamos la propiedad de almacenamiento en caché de los pipelines para almacenar en caché el gráfico de vecinos más cercanos entre múltiples ajustes de KNeighborsClassifier. La primera llamada es lenta ya que calcula el gráfico de vecinos, mientras que las siguientes son más rápidas ya que no necesitan volver a calcular el gráfico. Aquí las duraciones son pequeñas dado que el conjunto de datos es pequeño, pero la ganancia puede ser más sustancial cuando el conjunto de datos crece, o cuando la cuadrícula de parámetros a buscar es grande.
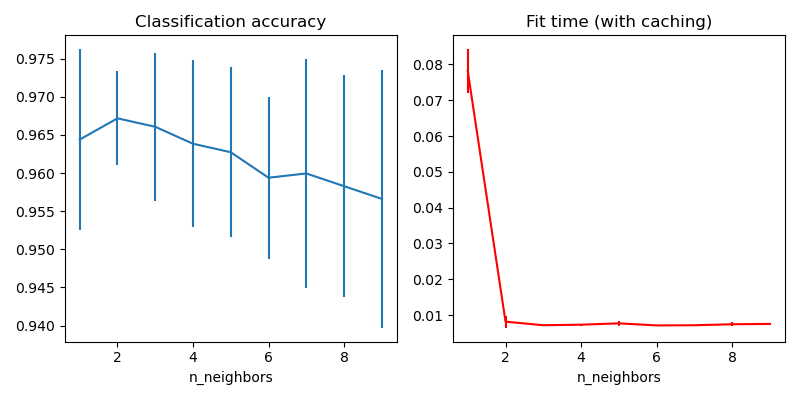
# Author: Tom Dupre la Tour
#
# License: BSD 3 clause
from tempfile import TemporaryDirectory
import matplotlib.pyplot as plt
from sklearn.neighbors import KNeighborsTransformer, KNeighborsClassifier
from sklearn.model_selection import GridSearchCV
from sklearn.datasets import load_digits
from sklearn.pipeline import Pipeline
print(__doc__)
X, y = load_digits(return_X_y=True)
n_neighbors_list = [1, 2, 3, 4, 5, 6, 7, 8, 9]
# The transformer computes the nearest neighbors graph using the maximum number
# of neighbors necessary in the grid search. The classifier model filters the
# nearest neighbors graph as required by its own n_neighbors parameter.
graph_model = KNeighborsTransformer(n_neighbors=max(n_neighbors_list),
mode='distance')
classifier_model = KNeighborsClassifier(metric='precomputed')
# Note that we give `memory` a directory to cache the graph computation
# that will be used several times when tuning the hyperparameters of the
# classifier.
with TemporaryDirectory(prefix="sklearn_graph_cache_") as tmpdir:
full_model = Pipeline(
steps=[('graph', graph_model), ('classifier', classifier_model)],
memory=tmpdir)
param_grid = {'classifier__n_neighbors': n_neighbors_list}
grid_model = GridSearchCV(full_model, param_grid)
grid_model.fit(X, y)
# Plot the results of the grid search.
fig, axes = plt.subplots(1, 2, figsize=(8, 4))
axes[0].errorbar(x=n_neighbors_list,
y=grid_model.cv_results_['mean_test_score'],
yerr=grid_model.cv_results_['std_test_score'])
axes[0].set(xlabel='n_neighbors', title='Classification accuracy')
axes[1].errorbar(x=n_neighbors_list, y=grid_model.cv_results_['mean_fit_time'],
yerr=grid_model.cv_results_['std_fit_time'], color='r')
axes[1].set(xlabel='n_neighbors', title='Fit time (with caching)')
fig.tight_layout()
plt.show()
Tiempo total de ejecución del script: (0 minutos 2.722 segundos)